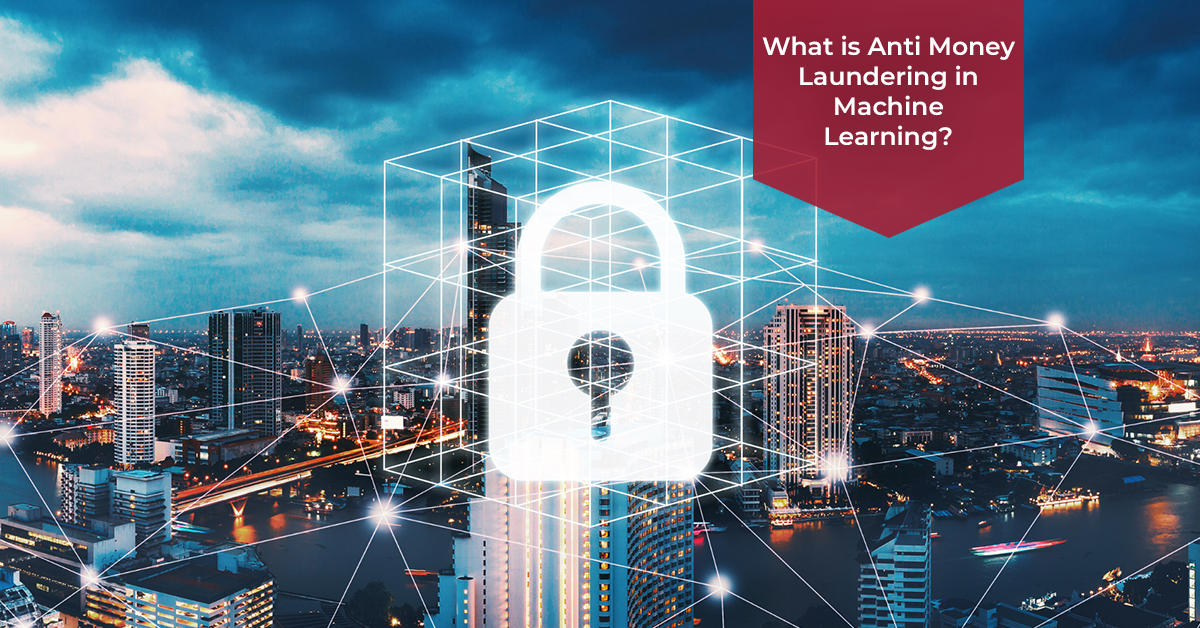
What is Anti Money Laundering in Machine Learning?
The worldwide increase in money laundering and financial crimes has led banks to invest substantially in bolstering their defenses. Nevertheless, traditional approaches face difficulties in keeping up with the constantly changing tactics criminals employ. The recent advancements in machine learning (ML) offer a pivotal opportunity for banks, particularly in enhancing transaction monitoring within anti-money laundering (AML) programs. Regulatory initiatives worldwide are increasingly supportive of these efforts. A recent industry discussion revealed a substantial interest among leaders to adopt ML solutions, signaling a noteworthy shift in the global approach to combating illicit financial activities.
How Transaction Monitoring Evolved with Machine Learning (ML)?
Harnessing the power of machine learning (ML) in transaction monitoring can revolutionize the entire Anti-Money Laundering (AML) value chain. While ML can be applied across various aspects of AML, combining it with advanced algorithms like random forest, gradient boosting, and deep learning in transaction monitoring stands out as an avenue where banks can achieve immediate and substantial benefits.
Traditional methods, such as rule- and scenario-based tools or basic statistical approaches, often fall short in capturing the latest money laundering trends. In contrast, ML models utilize more detailed, behavior-indicative data to develop sophisticated algorithms, offering greater flexibility in adapting to new trends and continually improving over time.
The adoption of machine learning models instead of traditional rule- and scenario-based tools by prominent financial institutions has led to notable advancements. Specifically, there has been a remarkable 40 percent improvement in the identification of suspicious activities, accompanied by a significant 30 percent increase in operational efficiency. This shift marks a substantial progression in the integration of machine learning within Anti-Money Laundering strategies and underscores the transformative impact on transaction monitoring processes.
How Banks Can Use Machine Learning (ML)?
Servicing machine learning (ML) models in banking poses challenges due to their inherent opacity. To address the demand for better model explainability from regulators and model risk management teams, leading institutions are adopting collaborative approaches. They work closely with AML investigators, ensuring understanding of modeling data, creating interpretable features, and integrating ML modules with existing rule-based models. This seamless transition enhances the status quo without dismantling it entirely. Key strategies include allocating sufficient testing samples, addressing ML-specific risks in model validation, and conducting ongoing monitoring for performance evaluation.
Best Methods in Transactions Monitoring with Machine Learning (ML)
Financial institutions integrating machine learning (ML) into Anti-Money Laundering (AML) transaction monitoring can follow three key best practices:
- Stakeholder Alignment: Involve various teams from the project’s start, including data, technology, line-of-business, model risk management (MRM), and compliance, to ensure agreement on vision and design. This enhances transparency, reduces risks, and improves ML’s effectiveness.
- Technology Transition Plan: Plan intentionally and execute rigorously. During the pilot phase, run existing risk scenarios alongside ML-based scenarios to gain early support and mitigate risks. Gradual integration and starting with low-risk projects contribute to a smoother adoption process.
- Model Risk Management Enhancement: Collaborate closely with data science teams throughout development and validation. Shape validation standards for ML-specific risks, including bias detection and explainability. Define precise performance and monitoring requirements to ensure continuous improvement and compliance.
Implementing these practices enables financial institutions to seamlessly integrate ML into transaction monitoring, enhancing risk detection and regulatory compliance.